MLOps to Tame Chaos and Innovate Faster
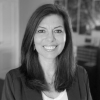
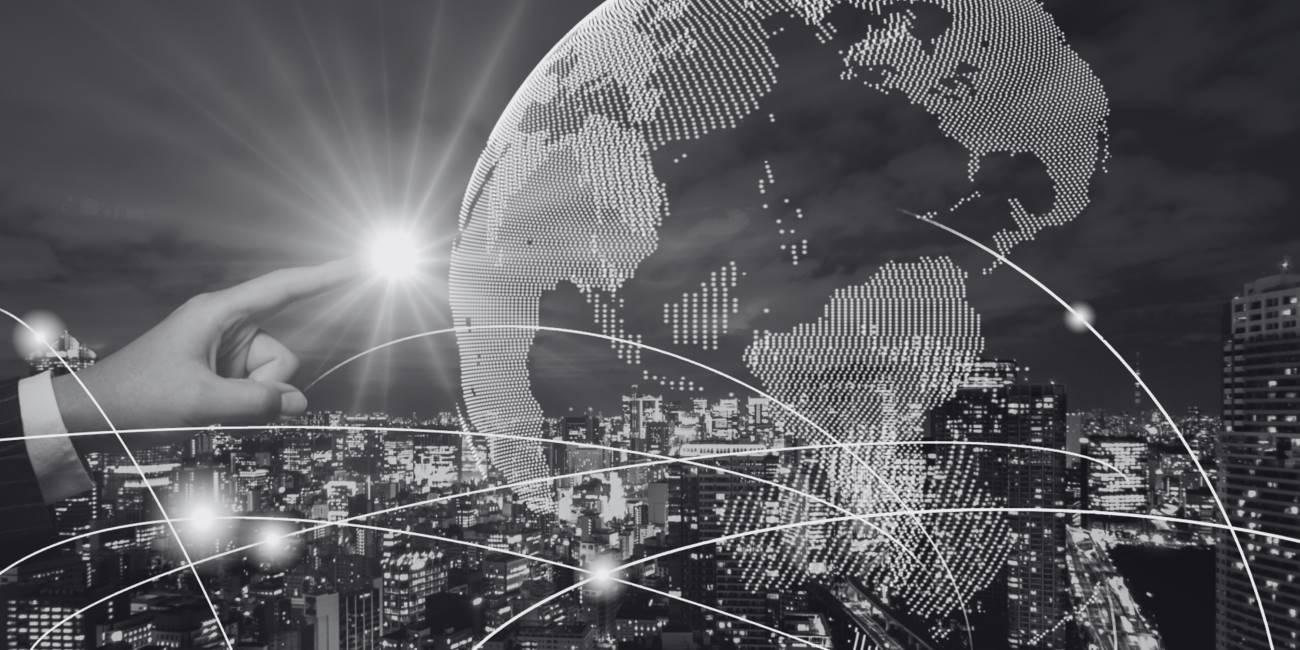
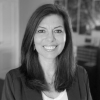
The Unprecedented Growth of AI and Machine Learning
Once the domain of niche research and the ambitious projects of large enterprise R&D teams, AI and MLOps now permeate every corner of our digital world. The release of OpenAI's DALL-E in early 2021 sparked a media frenzy, propelling generative AI into the limelight of popular culture and signaling a new dawn for AI innovation. This enthusiasm only grew with the launch of ChatGPT in late 2022, which astonishingly attracted 1 million users in just five days, smashing previous records and underscoring the growing public interest in AI technologies.
By early 2024, with ChatGPT's availability in 161 countries and a user base of 180.5 million, the evidence was clear: we were witnessing a new era of innovation and investment in AI and Machine Learning. The proliferation of open-source libraries and a Kaggle user base that skyrocketed to over 17 million in a decade exemplify the vibrant growth and development of AI solutions.
Amidst this growth, many companies have ventured into creating new Large Language Models (LLM) and ML solutions, diving headfirst into the experimental and fast-paced nature of AI field advancements. However, this rapid development often leads to operational chaos, underscoring the need for structured data management and experimentation practices.
The Critical Role of Data Management
According to IDC's AI Strategies View, about 21% of the AI/ML application lifecycle is dedicated to collecting and preparing data—a task that includes gathering, cleansing, augmenting, and normalizing data, among other processes. In an environment where the demand for ML and LLM services is skyrocketing, optimizing these processes for scalability is more important than ever.
Ensuring Data Compliance and Efficiency
The challenge of managing data effectively is compounded when ML teams collaborate with third parties for data labeling or software development. Without proper data handling protocols, data can become scattered across multiple locations, potentially violating data management policies and risking data loss without backups.
Tracking Data Through Its Lifecycle
From collection to cleansing, it's vital to maintain versions of data that AI and ML teams can utilize for model generation. Data may originate from diverse sources, presenting various formats and quality issues. The ability to trace back to the original data sets used in model training is crucial, especially when unexpected results arise, necessitating a reevaluation of the data.
Managing Experimentation and Model Development
Machine Learning thrives on experimentation. Organized approaches to managing experiments, including tracking results, algorithms, hyperparameters, and the data sets used for training, validation, and testing, are essential for refining models and achieving optimal outcomes.
The Introduction of MLOps Platforms and Tooling
As teams strive to keep pace with the rapid advancements in AI, the introduction of MLOps platforms like MLflow is a game-changer for development organizations. MLflow, for instance, offers a comprehensive solution for managing the machine learning lifecycle, encompassing experimentation, reproducibility, and deployment. Features such as experiment management, parameter and metric logging, artifact logging, and model registration and versioning enable teams to streamline their workflows, ensuring consistency, efficiency, and the ability to build upon previous successes.
Conclusion: Embracing Order in the Midst of Chaos
The journey of AI from niche curiosity to mainstream powerhouse is one of the most compelling narratives of modern technology. As we continue to push the boundaries of what's possible with AI and Machine Learning, the importance of structured data management, efficient experimentation practices, and the supportive role of MLOps platforms cannot be overstated. By embracing these tools and methodologies, we can navigate the complexities of AI development with confidence, paving the way for continued innovation and success in this exciting field.